Mental disorder is one of the leading causes of disabilities, diseases, and death around the world. Among many diagnoses, schizophrenia, a debilitating psychotic disorder characterized by significant impairments in cognition, perception, and behaviors towards reality, has a high mortality rate that affects around 0.32% of the population. Without a known cure, it is clinically crucial for patients to be diagnosed at its earliest onset, which would not only improve the treatment outcome but also lessens the burden on patients. Once diagnosed, patients with schizophrenia go through a range of treatment and care options that can help patients maintain their daily lives, such as medication, cognitive-behavioral therapy, and psychosocial rehabilitation. Moreover, around 80% of patients experience an exacerbation of symptoms, often called relapse, throughout the course of illness. Due to this chronic, disabling nature of schizophrenia, its symptoms, thus, should be constantly under-watched and monitored by both clinicians and patients themselves to detect the early signs of relapses and intervene to treat them. However, due to misconceptions and misunderstandings about the disorder, treatment is often sought after a long delay, which imposes more suffering and worsens the illness progression.
To address these challenges in mental health consultation and limitations in accurately monitoring and predicting patients' symptoms, clinicians and HCI researchers have sought new avenues of innovative technology-based solutions, using machine learning, digital health interventions, and passive sensing computing. In the context of technological endeavors, research has shed light on the potential of social media as a tool for mental health assessment and prediction. With a predictive modeling strategy based on a machine learning model, social media provides a large amount of users' real-time information about mental health in rich detail and context to assess their symptoms.
Despite the continuous research and achievements in utilizing AI technologies in mental health treatment, these tools are rarely being adopted in real clinical practices due to the unnecessary burden of dealing with false positives, some biases, and concerns about their possible negative impact on treatment outcomes. I want to explore the opportunity of social media-powered AI to be incorporated into clinicians' workflow as Clinical Decision Support Systems (CDSS) regarding relapse-related decisions and patient's agency in symptoms monitoring.
Using the human-centered design approach, I aim to conduct a co-design workshop by bringing patients with schizophrenia and clinicians to the table. I hope to derive design implications from the workshop that can inform design decisions to develop implementable and sustainable high-fidelity prototypes of social media data-driven patients-facing and clinician-facing tools that support both patients' narratives and clinician's workflow in making clinical decisions. With the social media-based relapse prediction model available from Dr. Munmun De Choudhury's previous work, I envision designing both website and mobile application prototypes based on the needs and goals determined during the co-design workshop. I expect a clinician-facing tool prototype to have features such as patient monitoring system based on their social media data sharing, social media-based relapse prediction prompts, and clinical decision suggestions. For the patient-facing tool prototype, I expect it to enable monitoring their own social media, making decisions on whether or not they want to share data with the clinicians, and receiving relapse predictions that facilitate the decision-making to make upcoming hospital visits.
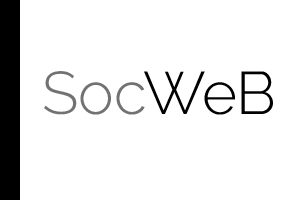
The SocWeB Lab's mission is to develop novel computational techniques, and technologies powered by these techniques, to responsibly and ethically employ social media in quantifying, understanding, and improving our mental health and well-being.